Table Of Content
- 3.1. 2x2 Factorial designs¶
- Selecting the Right Factors and Components in a Factorial Design: Design and Clinical Considerations
- 3.3. Interactions¶
- Contrasts, main effects and interactions
- Book traversal links for Lesson 5: Introduction to Factorial Designs
- How to Deal with a 2n Factorial Design
- Interaction Effects
- Achieving the Right Component Comparisons
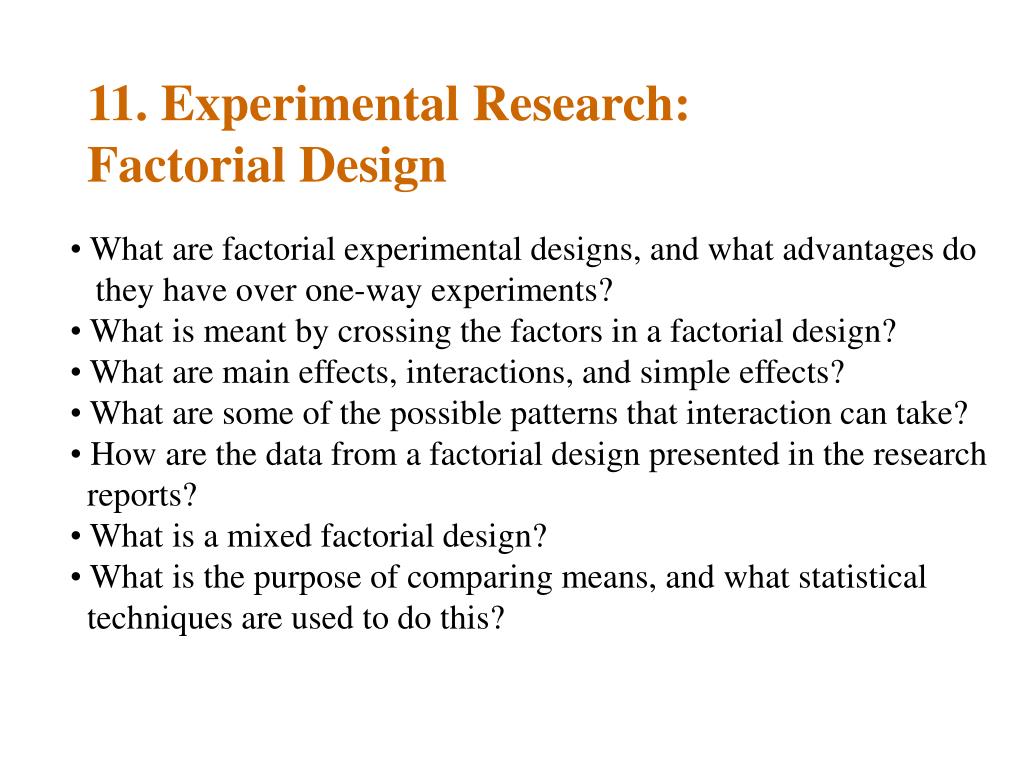
Finally, the researchers asked participants to rate their current level of disgust and other emotions. The primary results of this study were that participants in the messy room were in fact more disgusted and made harsher moral judgments than participants in the clean room—but only if they scored relatively high in private body consciousness. The number of ICs may affect the clinical relevance and generalizability of the research findings. Increased numbers of ICs and assessments may create nonspecific or attentional effects that distort component effects. For instance, while a real world application of a treatment might involve the administration of only two bundled ICs (counseling + medication), a factorial experiment might involve 6 or more ICs. Such effects would be manifest in interactions amongst components (e.g., the effectiveness of a component might be reduced when it is paired with other components) or in increased data missingness.
3.1. 2x2 Factorial designs¶
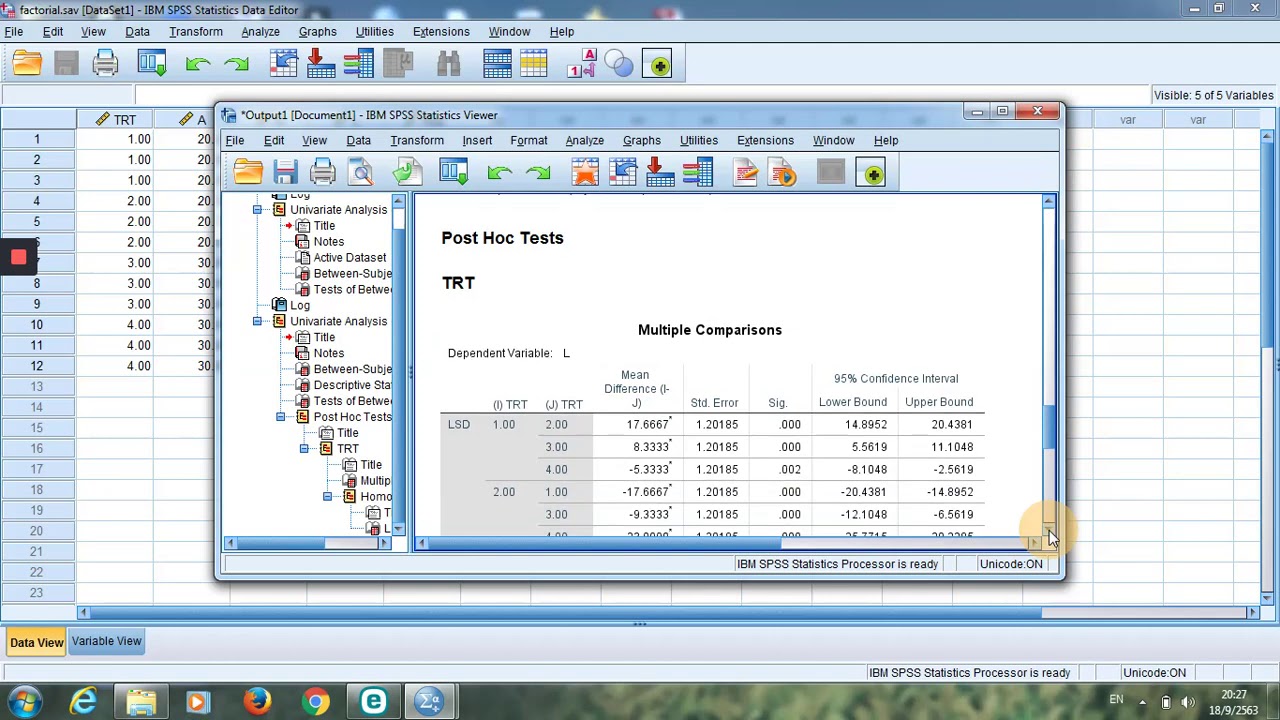
Main effects occur when the levels of an independent variable cause change in the measurement or dependent variable. There is one possible main effect for each independent variable in the design. When we find that independent variable did influence the dependent variable, then we say there was a main effect. When we find that the independent variable did not influence the dependent variable, then we say there was no main effect. The different ICs when used in real world settings would entail different amounts of contact or different delivery routes and their net real world effects would reflect these influences.
Development of ivermectin orally disintegrating tablets using factorial design: In-vitro evaluation and in vivo absorption ... - ScienceDirect.com
Development of ivermectin orally disintegrating tablets using factorial design: In-vitro evaluation and in vivo absorption ....
Posted: Thu, 13 Jul 2023 07:55:00 GMT [source]
Selecting the Right Factors and Components in a Factorial Design: Design and Clinical Considerations
Advice on "Lack of fit" and "replicate" points for an I Optimal Design of Experiments? - ResearchGate
Advice on "Lack of fit" and "replicate" points for an I Optimal Design of Experiments?.
Posted: Mon, 16 May 2022 07:00:00 GMT [source]
In other words, the effect of wearing a shoe does not depend on wearing a hat. More formally, this means that the shoe and hat independent variables do not interact. It would mean that the effect of wearing a shoe on height would depend on wearing a hat.
3.3. Interactions¶
But a multiple regression analysis including both income and happiness as independent variables would show whether each one makes a contribution to happiness when the other is taken into account. Research like this, by the way, has shown both income and health make extremely small contributions to happiness except in the case of severe poverty or illness [Die00]. Again, because neither independent variable in this example was manipulated, it is a correlational study rather than an experiment (the study by MacDonald and Martineau [MM02] was similar, but was an experiment because they manipulated their participants’ moods). This is important because, as always, one must be cautious about inferring causality from correlational studies because of the directionality and third-variable problems. For example, a main effect of participants’ moods on their willingness to have unprotected sex might be caused by any other variable that happens to be correlated with their moods. Manipulation checks are usually done at the end of the procedure to be sure that the effect of the manipulation lasted throughout the entire procedure and to avoid calling unnecessary attention to the manipulation.
Contrasts, main effects and interactions
Schnall and her colleagues also demonstrated an interaction because the effect of whether the room was clean or messy on participants’ moral judgments depended on whether the participants were low or high in private body consciousness. If they were high in private body consciousness, then those in the messy room made harsher judgments. If they were low in private body consciousness, then whether the room was clean or messy did not matter. Ambitious, multifactor, factorial experiments designed to evaluate clinical ICs can and do work for the purpose of intervention component screening (Baker et al., 2016; Collins et al., 2016; Collins, Murphy, & Strecher, 2007; Fraser et al., 2014). We believe that their potential to yield unique data, and to do so efficiently, should make factorial screening experiments a core strategy in the process of developing effective treatments (Collins et al., 2016).
Now we are going to shift gears and look at factorial design in a quantitative approach in order to determine how much influence the factors in an experiment have on the outcome. In the 2 × 3 example above, the degrees of freedom for the two main effects and the interaction — the number of columns for each — are 1, 2 and 2, respectively. Nature, he suggests, will best respond to "a logical and carefully thought out questionnaire". A factorial design allows the effect of several factors and even interactions between them to be determined with the same number of trials as are necessary to determine any one of the effects by itself with the same degree of accuracy. A design which manipulates one independent variable between subjects and another within subjects. A fractional design is a design in which experimenters conduct only a selected subset or "fraction" of the runs in the full factorial design.
How to Deal with a 2n Factorial Design
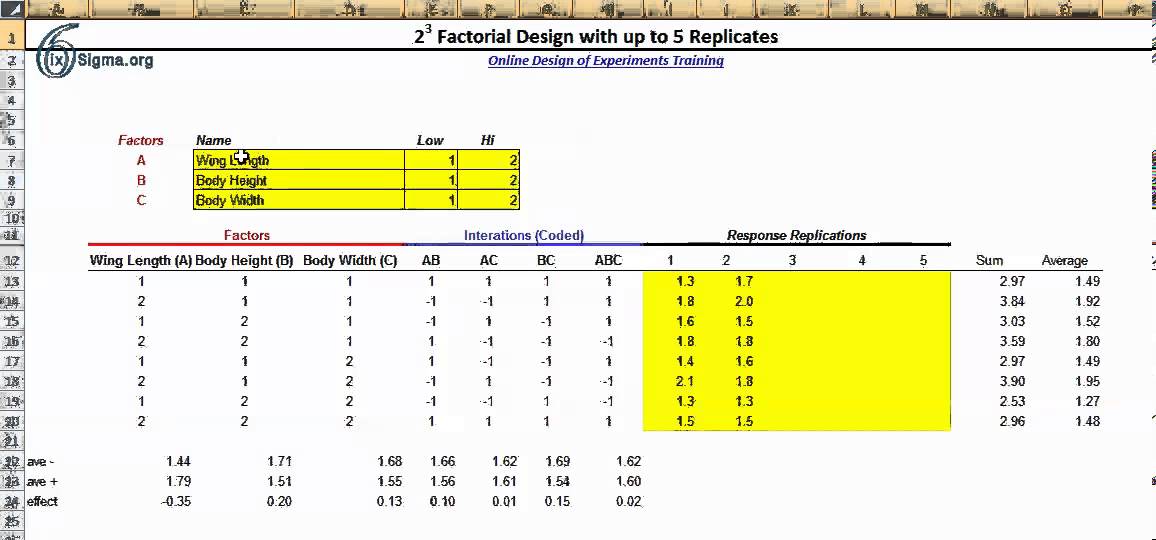
Factorial designs can pose challenges, but they offer important advantages that can offset such challenges. Of course, there is increased efficiency as investigators can screen more components at a reduced expenditure of resources. Thus, investigators must decide if they wish to directly compare two treatment conditions (and these may be multicomponential) with one another, without the results being affected by the presence of other experimental factors being manipulated. Again, because neither independent variable in this example was manipulated, it is a non-experimental study rather than an experimental design. This is important because, as always, one must be cautious about inferring causality from non-experimental studies because of the threats of potential confounding variables.
Interaction Effects
However, there may be situations when a design contains points that are impractical to conduct and choosing an appropriate fraction can avoid these points. By doing this, psychologists can see if changing the independent variable results in some type of change in the dependent variable. The quantities b1, b2, and so on are regression weights that indicate how large a contribution an independent variable makes, on average, to the dependent variable. Specifically, they indicate how much the dependent variable changes for each one-unit change in the independent variable.
Both of these graphs only contain one main effect, since only dose has an effect the percentage of seizures. Whereas, graphs three and four have two main effects, since dose and age both have an effect on the percentage of seizures. An interaction is a result in which the effects of one experimental manipulation depends upon the experimental manipulation of another independent variable. After you become comfortable with interpreting data in these different formats, you should be able to quickly identify the pattern of main effects and interactions.
The other was private body consciousness, a variable which the researchers simply measured. Another example is a study by Halle Brown and colleagues in which participants were exposed to several words that they were later asked to recall [BKD+99]. Some were negative, health-related words (e.g., tumor, coronary), and others were not health related (e.g., election, geometry). Results from this study suggested that participants high in hypochondriasis were better than those low in hypochondriasis at recalling the health-related words, but that they were no better at recalling the non-health-related words. In many factorial designs, one of the independent variables is a non-manipulated independent variable. Some were negative health-related words (e.g., tumor, coronary), and others were not health related (e.g., election, geometry).
This framework can be generalized to, e.g., designing three replicates for three level factors, etc. The columns for AB, AC and BC represent the corresponding two-factor interactions. The columns for A, B and C represent the corresponding main effects, as the entries in each column depend only on the level of the corresponding factor. For example, the entries in the B column follow the same pattern as the middle component of "cell", as can be seen by sorting on B.
Each cluster is then interpreted as multiple measures of the same underlying construct. The Big Five personality factors have been identified through factor analyses of people’s scores on a large number of more specific traits. For example, measures of warmth, gregariousness, activity level, and positive emotions tend to be highly correlated with each other and are interpreted as representing the construct of extraversion. As a final example, researchers Peter Rentfrow and Samuel Gosling asked more than 1,700 university students to rate how much they liked 14 different popular genres of music [RG03]. They then submitted these 14 variables to a factor analysis, which identified four distinct factors. Most complex correlational research, however, does not fit neatly into a factorial design.
No comments:
Post a Comment